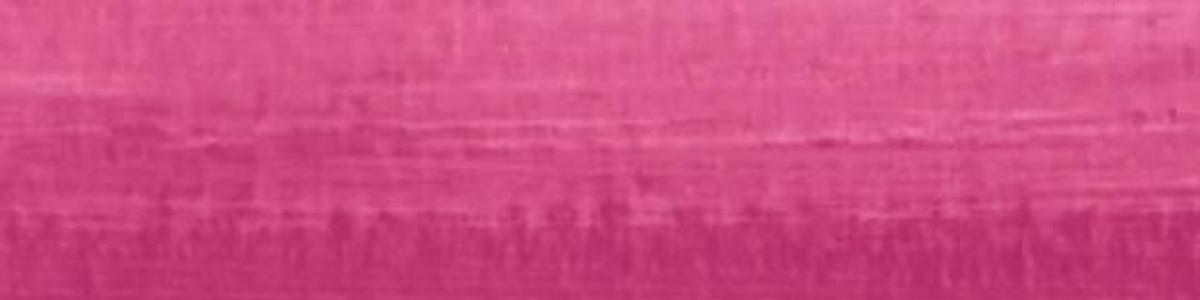
The UK government’s exam results debacle: questioning the reliability of algorithms
This year, Gavin Williamson, the British education secretary, chose to use an algorithm to award exam results during the pandemic. Students were exempted from taking exams and waited for results based on the teacher evaluation then corrected by an independent organism (Ofqual) on statistical criteria, including the past performance of schools. The results fell at the end of August: nearly 40% of teacher assessments were lowered, especially in the most deprived neighbourhoods.
"I am afraid your grades were almost derailed by a mutant algorithm and I know how stressful that must have been", the Prime Minister Boris Johnson told pupils at a school. His government made a complete turnaround following anger over the algorithm and decided to use grades from teachers instead.
This puts into sharp focus how algorithms and artificial intelligence can embed biases. Most people think using an algorithm can reduce bias in process by removing the element of human judgement. However, we are still at the beginning of this form of technology. Kim Nilsson, CEO of data science company Pivigo, explains biases interfere in algorithms because those are machines built on inherently human decisions.
"There is a real risk to perpetuate stereotypes and racism", Kim Nilsson says
Nimmi Patel, policy manager for skills, talent and diversity at industry body TechUK, emphasizes the urgency of the situation. "If we continue to develop AI models without taking out historical race and gender bias, systems will perpetuate stereotypes and racism. These two data scientists have two ideas to mitigate this :
- The diversity of teams developing AI models has to be improved in order to ensure the fairness of any algorithm. Biases appear when some groups are overrepresented in datasets and underrepresented in the team building the algorithm.
- Biases in data must be removed when they are identified to train algorithmic decision-making. Some technology companies have introduced advisory boards to assess technology and monitor algorithms development. The Partnership on AI in 2016 had reunited Google, Facebook and Apple to enhance research on the ethics of AI.
Another issue is the lack or absence of data scientists training about ethics and bias. Nilsson mentions we need to invest more in educating new entrants to the industry. Finally, to remove bias and discriminatory attitudes from our society requires more work than simply AI models. As technology becomes more and more entrenched in our lives, this tool has to be used in the right way and very carefully.
Pour La Solidarite-PLS advocates for a technology that contributes to social inclusion and not to the reinforcement of discriminations !